Can Machine Learning Help Us Measure the Trustworthiness of News?
Quality, fact-based news and trust between citizens and journalists are essential to helping people make informed decisions about important issues.
Traditional methods of evaluating media content are resource-intensive and time-consuming, so we tested whether machine learning can help catch news articles that contain journalists’ opinions and biases.
The experiment
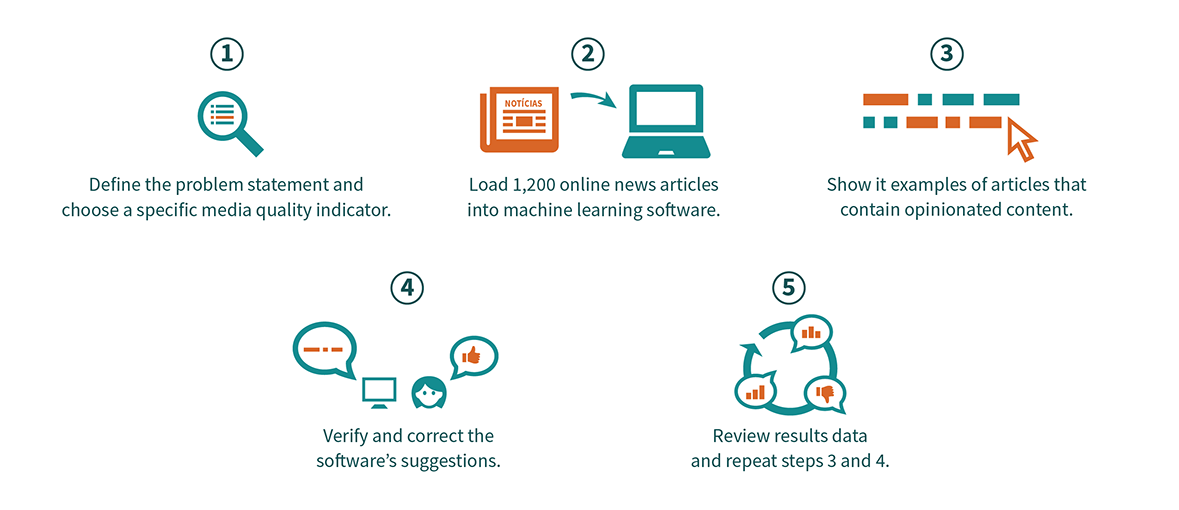
The team used software to scan websites for nine leading print media outlets and imported more than 1,200 articles into the machine learning software.
The evaluators then began training the tool to identify opinions in the text. Using the software’s “highlighter” tool, evaluators clicked sentences in the articles to show the software examples of opinions. Using these examples as a guide, the software identified patterns and searched for other sentences that were similar.
Evaluators reviewed sentences that the software flagged as possible opinions and determined whether the suggestion was correct or incorrect. The team conducted this feedback loop 51 times.
Key findings
This experiment tested whether software can automatically evaluate the impartiality of online news articles, specifically by identifying opinions in news articles. The results prove that it is feasible for machine learning to help us find opinions in news articles. The software identified these articles reliably enough to apply it to monitoring and evaluating media content, at a scale and speed that far outpace conventional human evaluators (a matter of seconds, instead of minutes or hours).
- 95% accuracy: The software found articles containing an opinion 95 out of 100 times.
- 84% precision rate: In other words, if the software thinks that 100 articles contain opinions, 84 of them actually do.
- 93% recall rate: Out of every 100 articles that actually contained opinions, the software found 93 of them but missed seven.
- Accuracy and precision increased the more that the model was trained: There is a clear relationship between the number of times the evaluators trained the software and the accuracy and precision of the results. The recall results did not improve over time as consistently.
- The software’s ability to “learn” was almost immediately evident: The evaluation team noticed a marked improvement in the accuracy of the software’s suggestions after showing it only 20 sentences that had opinions.
Lessons and limitations
- This experiment tested only one of IREX’s 18 indicators of media quality, the indicator about whether the author inserts their own opinion into articles. Others indicators, like whether the article cites a variety of reliable sources, are not as easy to automate.
- As in many machine learning applications, human bias can become codified in the software. Measuring media quality can be a subjective exercise. This software doesn’t eliminate bias, but it does apply it more consistently.
- More research and experimentation is necessary. Machine learning can help us spend resources more efficiently, but more exposure to the technology is needed to realize its potential appropriately and responsibly.
For more information, contact cali@irex.org.
This partnership between IREX and Lore.AI was supported by IREX’s Center for Applied Learning and Impact and tested in the Mozambique Media Strengthening Program (MSP), funded by the United States Agency for International Development.